
Underwater wireless network of Autonomous Underwater Vehicles (yellow vessels with blue fins) and anchored sensors (red).

Figure 1 shows a sketch of an underwater wireless sensor network that is represented by a swarm of mobile and static nodes. The locations of individual nodes must be known and tracked during operation in order to successfully complete assigned missions. In a sub-sea mission, a swarm of mobile and/or static nodes is typically deployed to communicate and collaboratively achieve various predefined tasks in underwater environments. The navigation module of an autonomous node estimates its position and velocity and then feeds them into the control and guidance modules. Localisation is one of the most critical problems in robotic swarms, as it is required to be successfully obtained in advance of nodes’ guidance and control.
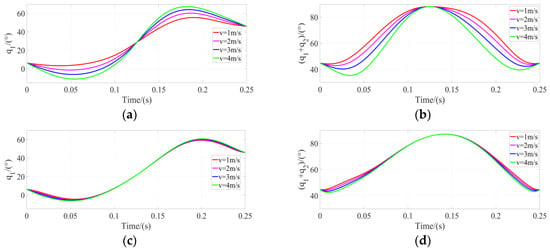
The wide variety of marine missions that can be achieved by means of mobile underwater sensor networks (i.e., underwater swarm robotics), such as deep sea exploration and environmental monitoring, have enabled and motivated underwater robotics research for decades. Underwater robotic swarm deployment is particularly challenging, due to the high cost of maritime assets and limited bandwidth of underwater acoustic communication channel. Radio and acoustic links are examples of direct communication, whereas indirect communication occurs through the environment, such as stigmergic collaboration. Intra-swarm communication can be achieved in either direct or indirect fashion. Swarm connectivity is a primary concern of any swarm system, which is realised by intra-swarm communication to enable nodes collaboration. While aerial and terrestrial swarm robotics have been extensively investigated, there has been little investigation of underwater robotic swarms. Over the past two decades, swarm robotics have been widely investigated and robotic swarms have been proven to be more efficient in solving complicated tasks or tasks that require wide spatial coverage than a single overly complicated robot. The proposed fuzzy-based localisation algorithm improves the entire swarm mean localisation error and standard deviation by 16.53% and 35.17%, respectively, when compared to the Extended Kalman Filter based localisation with round-robin scheduling.

A physics-based simulation platform that considers environment’s hydrodynamics, industrial grade inertial measurement unit, and underwater acoustic communications characteristics is implemented in order to validate the proposed localisation framework on a swarm size of 150 autonomous underwater vehicles. Simplicity, flexibility, and scalability are the main three advantages that are inherent in the proposed localisation framework when compared to other traditional and commonly adopted underwater localisation methods, such as the Extended Kalman Filter. The proposed navigation framework harnesses the established localisation methods in order to provide navigation aids in the absence of acoustic exteroceptive sensors navigation aid (i.e., ultra-short base line) and it can be extended to accommodate newly developed localisation methods by expanding the fuzzy rule base. A number of underwater localisation methods have been proposed in the literature for wireless sensor networks. This article proposes a holistic localisation framework for underwater robotic swarms to dynamically fuse multiple position estimates of an autonomous underwater vehicle while using fuzzy decision support system.
